Have you ever wondered if that perfectly-written essay was created by a human or an AI? AI detectors are tools designed to answer exactly that question. They work by analyzing text patterns that differ between human and AI-generated content. AI detectors work by examining specific characteristics in text, such as word choice patterns, sentence length variations, and the overall predictability or randomness of the writing style.
These detection systems use statistical models that have been trained on thousands of examples of both human and AI writing. When an AI like ChatGPT writes content, it tends to follow certain predictable patterns that humans don’t. For instance, AI often uses less random word choices and more consistent sentence structures than humans who naturally vary their writing style.
While these detection tools have become increasingly sophisticated, they aren’t perfect. They sometimes struggle with highly edited AI text where many words have been changed and sentences restructured. This cat-and-mouse game between AI generators and AI detectors continues to evolve as both technologies advance.
Key Takeaways
- AI detectors analyze text for patterns like predictability in word choice and sentence structure to identify AI-generated content.
- These detection tools compare text against statistical models trained on examples of both human and artificial writing.
- No AI detector is 100% accurate, with effectiveness varying based on text length, editing, and the specific AI system that generated the content.
Understanding AI Detectors
AI detectors serve as digital tools that analyze content to determine if it was created by artificial intelligence or humans. These systems rely on specific patterns and characteristics found in AI-generated text to make their determinations.
Definition and Purpose
AI detectors are specialized tools that analyze text to identify if it was written by an AI system rather than a human. They examine linguistic patterns, sentence structures, and word choices that might indicate machine generation. The primary purpose of these detectors is to maintain content authenticity and prevent potential misuse of AI-generated content.
These tools have become increasingly important as AI writing systems like ChatGPT have become more sophisticated. Schools use them to check for academic integrity in student assignments. Publishers employ them to verify original human content. Businesses utilize them to ensure authentic marketing materials.
The reliability of these detectors varies, with most offering probability scores rather than definitive answers about content origin.
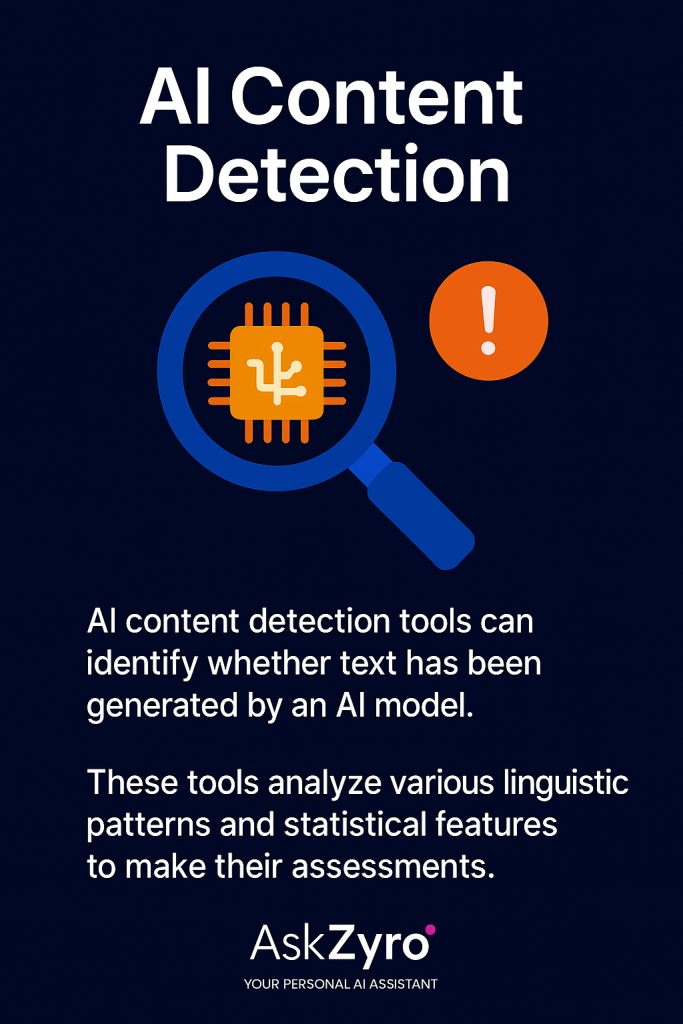
Types of AI Detectors
Several approaches exist in AI detection technology. Statistical-based detectors measure specific characteristics of text like perplexity (randomness of word choices) and burstiness (variation in sentence structure). These metrics often differ between human and AI writing.
Pattern recognition detectors focus on identifying repetitive structures or predictable language that AI tends to produce. They look for telltale signs like formulaic transitions or consistently balanced paragraph lengths.
Machine learning detectors use trained algorithms that have studied millions of text samples. These systems can spot linguistic patterns invisible to humans by analyzing subtle features in text organization.
Some advanced detectors combine multiple approaches for higher accuracy, though no detector is 100% reliable. New AI models constantly evolve to produce more human-like text, creating ongoing challenges for detection technology.
Mechanisms of Detection
AI detectors use several technical approaches to identify artificially created content. These systems analyze text characteristics, look for patterns common in AI writing, and detect anomalies that differ from human writing styles.
Machine Learning Models
AI detectors use similar language models to the AI writing tools they’re designed to identify. These models are trained on vast datasets containing both human-written and AI-generated content.
When analyzing text, the detector evaluates whether the content resembles something its own model would produce. This comparison helps establish a probability score for AI generation.
Most effective detectors employ sophisticated neural networks that can recognize subtle linguistic features unique to AI systems. These features include word choice patterns, sentence structure consistency, and predictable language usage.
We find that detectors often use transformer-based architectures similar to those in GPT models but optimized specifically for detection rather than generation.
Pattern Recognition
AI content often contains recognizable patterns that differ from human writing. Detectors analyze these patterns at multiple levels – word choice, sentence structure, and overall text flow.
Statistical analysis plays a key role in identifying repetitive phrasing or unnaturally consistent language that AI tends to produce. For example, AI writing might use certain transition words too frequently or maintain overly consistent sentence lengths.
The detection algorithms also examine semantic coherence and how ideas connect throughout the text. AI-generated content might display unusual patterns in how topics develop or fail to include the natural variations typical in human writing.
We can observe that pattern recognition algorithms in detectors focus particularly on stylistic consistency that exceeds what would be normal in human writing.
Anomaly Detection
Anomaly detection identifies unusual characteristics that distinguish AI content from human writing. These systems look for statistical outliers in text properties that typically indicate machine generation.
One key approach examines perplexity and burstiness. Perplexity measures how predictable text is, while burstiness captures the natural variation in human writing. AI-generated text often shows lower perplexity and burstiness scores than human writing.
Detectors also analyze linguistic features like:
- Emotional consistency
- Cultural references
- Personal experiences
- Informal language patterns
We’ve observed that sophisticated detectors can identify when content lacks the natural irregularities and imperfections characteristic of human writing. These systems continuously improve as they analyze more examples of both AI and human text.
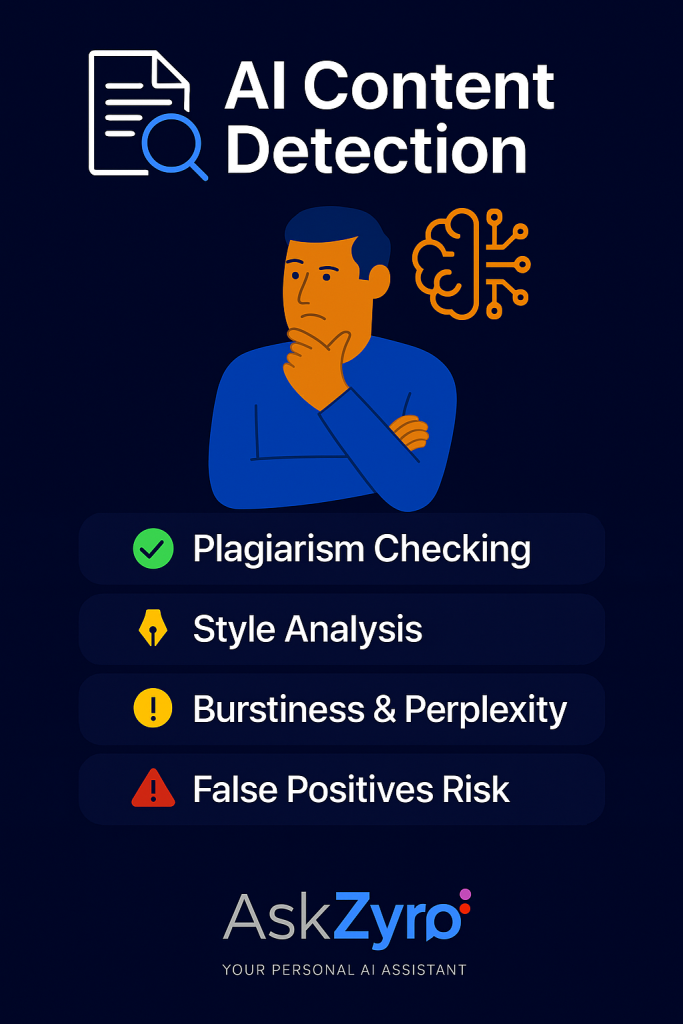
Applications and Use Cases
AI detectors serve important functions across different industries. They help organizations identify AI-generated content and protect against potential misuse while maintaining quality standards.
Content Moderation
AI detectors play a crucial role in content moderation for online platforms. Social media companies use these tools to identify potentially AI-generated posts that might spread misinformation. Educational institutions employ detectors to ensure academic integrity by flagging assignments that may have been created using AI writing tools.
News organizations and publishers also benefit from these systems to verify the authenticity of submitted articles. This helps maintain journalistic standards in an era where AI can produce convincing text.
Many content platforms now integrate AI detection as part of their quality control process. This allows them to label or filter content appropriately based on its origin. The goal isn’t always to block AI content but to create transparency for users.
Security and Fraud Prevention
In security contexts, AI detectors help identify automated content that might be part of phishing campaigns or social engineering attacks. Financial institutions use these tools to flag suspicious communications that might be machine-generated attempts at fraud.
Customer service departments implement detection systems to identify when they’re interacting with bots rather than humans. This helps prioritize genuine customer inquiries and maintain service quality.
Legal and compliance teams use AI detection to verify the authenticity of documents. This is particularly important in contexts where forged or artificially generated content could have legal implications.
Insurance companies employ these tools to analyze claim descriptions for potential fraud indicators, including text that might have been generated to create false claims.
Challenges and Limitations
AI detectors face significant hurdles that impact their reliability and ethical use. These tools struggle with accuracy issues and raise important concerns about fairness and privacy in educational settings.
Accuracy Concerns
AI detectors frequently produce false positives, incorrectly flagging human-written content as AI-generated. This happens because they analyze text patterns that can appear in both human and AI writing.
The accuracy rates vary widely across different detection tools. Many current detectors achieve only 60-80% accuracy rates in controlled tests, making them unreliable for high-stakes decisions.
These tools often struggle with specific limitations that affect their performance:
- Language complexity: More sophisticated AI outputs can easily bypass detection
- Text length: Short submissions provide insufficient data for accurate analysis
- Paraphrased content: Minor edits to AI text can defeat many detectors
Even advanced detectors can be fooled by simple techniques like changing sentence structure or using synonyms.
Ethical Considerations
Using AI detectors raises serious ethical concerns about fairness and justice. When institutions rely on error-prone tools, students may face wrongful accusations of academic dishonesty.
These false accusations disproportionately affect non-native English speakers and students with unique writing styles. Their legitimate work may be flagged simply because it differs from expected patterns.
Privacy issues also emerge when student work is processed through third-party detection services. We must consider who owns and has access to this data after submission.
The implementation of these tools can negatively impact student well-being by creating an atmosphere of surveillance and mistrust. This environment may inhibit creativity and authentic expression.
Frequently Asked Questions
AI detection technology uses specific methods to identify machine-generated content. These tools analyze text patterns, language structures, and statistical indicators to distinguish between human and AI writing.
What techniques are used to identify AI-generated content?
AI detectors examine burstiness and perplexity in text. Perplexity measures how predictable the text is, while burstiness looks at variations in sentence structure.
These tools also analyze linguistic patterns unique to AI writing. They check for repetitive phrasing, consistent tone, and unnaturally perfect grammar.
Many detectors use machine learning models similar to those that generate AI text. They essentially ask: “Is this something I would have written?”
How can AI detectors differentiate between human and AI-written essays?
Humans typically write with more variation in sentence structure and word choice than AI. AI detectors identify these statistical differences.
Human writing often contains unexpected transitions, quirky phrases, and personal anecdotes. AI writing tends to be more formulaic and consistent.
We’ve found that detectors also look for patterns in complexity levels throughout a document. Humans naturally vary their complexity, while AI may maintain a more consistent level.
What is the accuracy rate of current AI detection tools?
Current AI detectors typically achieve accuracy rates between 70-90% under ideal conditions. This varies widely based on the specific tool and type of content.
Shorter texts are generally harder to analyze accurately. We’ve seen that texts under 300 words often result in less reliable detection outcomes.
Academic content detection tends to be more accurate than creative writing detection. The structured nature of academic writing makes patterns easier to identify.
Can AI content detectors be fooled or bypassed?
Yes, AI detectors can be fooled using various techniques. Specialized prompts can generate text that evades many detection systems.
Manual editing of AI-generated text often reduces detectability. Adding personal anecdotes, intentional errors, or unique phrasing makes content appear more human-like.
Tools specifically designed to “humanize” AI text exist and can reduce detection rates. However, these tools often reduce content quality in the process.
In what ways might AI content detectors produce false positives?
AI detectors may flag human-written content as AI-generated when it follows formal structures or uses technical language. Academic and legal writing are particularly vulnerable.
Non-native English speakers often receive false positives. Their writing patterns can sometimes resemble AI-generated text due to learned formality.
Highly edited or professionally polished content tends to trigger false positives. The reduction of natural language quirks can make human writing appear machine-like.
What advancements are being made in the field of AI detection technology?
Researchers are developing more sophisticated algorithms that can identify subtle linguistic markers unique to different AI writing systems.
Multi-modal detection systems are emerging that analyze both text content and metadata. These systems examine factors beyond just the words themselves.
We’re seeing progress in contextual analysis that examines how ideas develop throughout a document. This helps distinguish between human thought progression and AI generation patterns.